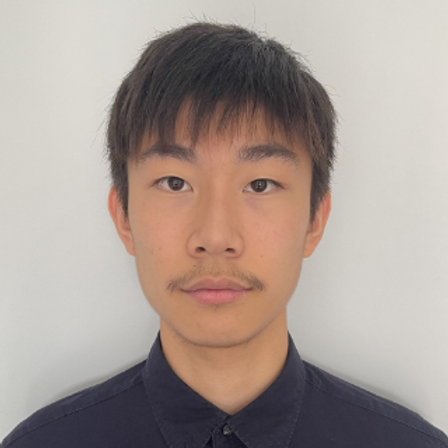
hello
I am liangchenyu
Hello, everyone, this is a brief introduction to everything about my past, I hope you can understand me better after reading.
Education
Ulink College of Shanghai 08/2021 – present | Shanghai, China Graduation Date: 06/2025 GPA: 4.0 / 4.0, Unweighted A-Levels Curriculum: Biology,Chemistry, Physics, Further Mathematics, Mathematics, English Literature, Chinese, Extended Project Qualification (EPQ) IGCSEs: English EFL, Chinese, Economics, Geography, Chemistry, Biology, Mathematics, Physics Standardized Tests: 1530 SAT; 110 TOEFL
Awards and Honors
Global Gold, British Biology Olympiad 2024 Global Gold, Chemistry Olympiad 2024 - UK Round One Global Gold, iGEM Competition 2023 National Gold, USA-CHINA BioOlympiad Initiative 2024 Distinction (Top 5%), American Mathematics Competition 12 2023 Distinction (Top 25%), Euclid Contest 2023 Scored 95/100, Top 1% Third Place, Ulink Poetry Competition
Research Experience
Ulink College of Shanghai, Independent Researcher 01/2024 – 05/2024 • Did research on pathways related to prostate cancer; • Wrote a 5000-word essay on epigenetic mechanisms and epi-drug feasibility; • Received an A* in iEPQ. iGEM, Team Ulink College of Shanghai, Wet Lab Co-Leader 03/2023 – 11/2023 • Built 3 PT-DNA-dependent enzymes; • Used 2 methods to purify & validate the product respectively; • Produced the team promotional and presentation video. Exploration and Determination of Lung Cancer Targets Using Multi-omics Approaches, Co-author and Researcher 02/2023 – 11/2023 • Standardized existing transcriptomic data; • Determined genes that are aberrantly expressed which could serve as cancer biomarkers; • Accepted by proceedings of 2nd International Conference on Modern Medicine and Global Health 2024.
Extracurricular Activities
Rangers Soccer Club, Varsity Soccer UCS, Midfielder 09/2022 – present • Played in league and cup games; • Competed with other schools for trophies and achieved 3rd place in SSSA; • Improved my fitness, stamina, and resilience. Extended Reading in Science, Reader 09/2021 – present • Sought extra reading in Campbell Biology, Immune by Kurzgesagt, What is Life, Developmental Biology, A Very Short Introduction; • Read more than 55 published papers out of pure interest. Enchanted Biology Club, Leader 09/2023 – 06/2024 • Organized 30+ weekly seminar sessions and practicals; • Set up 3 experimental protocols; • Gained the skills to become a mentor; advised 4 peers on EPQ sources. BioChem Terminator, a Bilibili Knowledge Sharing Channel, Content Creator 02/2024 – 06/2024 • Published my thoughts on past paper questions on my channel to aid the preparation of viewers; • Created 14 videos, and gained 300+ followers and 46,000+ views. Poetry Club, Member 10/2022 – 04/2023 • Discussed elements such as meters and rhymes and connecting the dictions with images; • Created our own pieces and organized seminars on our favorite poets.
Community Service
UCS Textbook Recycling Program, Founder & Volunteer 05/2022 – 06/2024 • Established the book recycling program to improve the use of the send-hand textbooks; • Put more than 160 textbooks in total to better use. Xiyanghong Assisted Living, Workshop Organizer & Lecturer 08/2024 – 10/2024 • Advertised and invited elders into seminars; • Discussed various topics ranging from healthy living guidances to nature of live and death. UCS Freshmen Week, Event Organizer 08/2023 • Researched and brainstormed about activity types to improve bonding; • Planned collaborative sport, construcion and treasure hunt activities for over 200 students. UCS Education Reform Program, Volunteer 02/2022 – 04/2022 • Translate 50 pages of a Chinese book on school transformation; • Crosschecked our work with other translators; • Assisted foreign faculty members at school in understanding new policies.
Skills
Bio Research
Snapgene ; Genetic Engineering; Experimental Design; Field Research;
Literature Review
Lab
PCR; Gel Electrophoresis; Dissection;Chromatography; Centrifugation
Quant
Data Analysis; Population Genetics Analysis
Language
Chinese; Native; English; Fluent
Music Composition
MuseScore
Certificates
Piano Performance - Level 8
Issuer: International Examination Board of Shanghai Conservatory of Music
Music Theory - Level 8
Issuer: International Examination Board of Shanghai Conservatory of Music
Certificate of merit.
Below are some of the awards I have won in the competitions I have participated in.
Can Epigenetic Engineering be Employed to Enhance the Effectiveness of Cancer Therapies Through Targeted Epigenetic Modifications
Introduction Cancer is a major global health concern, responsible for approximately 10 million deaths in 2020, representing nearly one-sixth of all deaths worldwide. In general, breast, lung, colorectal, and prostate cancers are among the most prevalent types worldwide. Cancer rises from the uncontrolled proliferation of mutated somatic cells. These cells have special features that allow them to reduce the period of the cell cycle and evade the immune system. Oncogenes are genes that lead to cancer. Originally found in viruses, many of its counterparts ended up in the human genome as proto-oncogenes, which promote normal growth and division of cells. Genetic alterations that promote the formation of oncogenes from proto-oncogenes belong to mainly four categories, those are epigenetic changes, point mutations, gene amplification and translocations. To begin with, the alteration of chromatin structure caused by epigenetic changes may serve to alter gene expression. For instance, acetylation by histone acetyltransferases loosens the chromosome and neutralises the charges on DNA to tune expression to an extremely high level. Such modification on a proto-oncogene would severely boost expression, turning it into an oncogene. On the other hand, point mutations alter one digit in the cell genome. For proto-oncogenes, a change within the promoter and enhancer region, which normally regulates expression, may alter gene regulation, causing it to be over-expressed and become an oncogene. Apart from slight changes in the genome, chromosomal translocation and duplication often rearrange a section of the chromosome. For example, translocation occurs when two sections of non-homologous chromosomes swap place, while duplication inserts a copy of an existing gene to that locus. In effect, if the translocated region contains a proto-oncogene and is placed near an active promoter, the genes are overexpressed; similarly, duplication causes gene expression to double which amplifies gene product. Namely, chronic myelogenous leukemia (CML) results from the translocation between chromosome 22 and 9 in white blood cells during mitosis, leading to uncontrolled cell cycle progression. Unlike genes, which are sequences encoded by nucleotides that form the chromosome by wrapping around histones. The epigenome is represented by modifications on the nucleotides and histones that do not alter the genetic sequence of the cell. Typical modifications associated with gene expression include DNA methylation and histone modifications. The two modifications differ in the location of the modification and their effects on transcription. DNA methylation is represented by the addition of methyl groups(-CH3) on the cytosine bases. The addition of methyl groups is carried out by DNA methyltransferases and its removal is catalysed by DNA demethylases. Overall, methyl groups are the promoter and enhancer regions that act as barriers that prevent the binding of protein complexes containing transcription factors and polymerases, thereby reducing transcription. They are frequently found in genes, especially the promoter regions, that are silenced or less expressed than others, as well as on inactivated X chromosomes in females. Unlike methylation which applies on the nucleotides, modifications are also applied on histone proteins, thanks to its unique histone tail that protrudes outward. Multiple organic groups are addible to the histone tail, including acetyl, methyl and phosphate groups. Generally, the addition of acetyl groups is found to neutralise the charge around histone molecules, leading to a loosened chromosome on which molecules can more easily bind and start transcription. The addition of acetyl groups and catalysed by histone acetyltransferases and its removal is catalysed by Histone deacetylases. Conversely, the addition of methyl groups on histones is observed to decrease transcription by tightening the chromosomes around histones, making it harder for transcription factors to bind to it. Compared to genetic mutations, epigenetic modifications are reversible by exposure to related enzymes, causing it to be a promising approach to alter high expression caused by cancer in a short period. Factors that increase the rate of mutation and promote cancer are called carcinogens, alcoholics, tobacco and air pollution are all known risk factors for oncogene formation. They account for approximately one-third of cancer-related deaths. Chronic infections also contribute to cancer risk, particularly in less economically developed countries (LEDCs). In 2018, approximately 13% of globally diagnosed cancers were linked to carcinogenic infections including hepatitis B virus (HBV), hepatitis C virus (HCV) and human papillomavirus (HPV). In LEDCs, infections like human papillomavirus (HPV) are responsible for about 30% of cancer cases. Effective detection and treatment can lead to successful cures for many cancers. Standard treatments for localized prostate cancer, stages I-III, include active surveillance, radical prostatectomy and radiotherapy. For men with recurrent prostate cancer, options include chemotherapy, radiotherapy, and androgen deprivation therapy (ADT). To begin with, active surveillance involves closely monitoring cancer with a plan to treat it if it shows signs of growth. It is often used for small, non-metastatic, or slow-growing cancers in the very low, low, or favourable intermediate-risk group. Benefits include retaining the normal function of the male gonad, lowering the cost of treatment, and avoiding unnecessary treatment. However, disadvantages include the risk of cancer spreading before treatment, missed opportunities for a cure, increased patient anxiety, and frequent medical checks. In addition, radiotherapy is a highly effective treatment for prostate cancer. Cancer cells are destroyed by high doses of radiation, delivered to the cancerous cells using techniques such as brachytherapy and external beam radiation therapy (EBRT). Brachytherapy involves transferring radioactive sources into the prostate gland. EBRT uses strong X-ray beams to target prostate tissues specifically. This technique outweighs surgical therapy in a significant aspect, as it often results in fewer risks such as bleeding, heart attack, blood clots, urinary incontinence, and erectile dysfunction. Furthermore, radical prostatectomy is the surgical removal of the prostate gland, performed through open or laparoscopic surgery. It is typically done for more severe cancer cases. Moreover, hormonal therapy, or androgen deprivation therapy (ADT), is used to treat advanced or metastatic prostate cancer. It works by blocking the production of testosterone and other male hormones, which can fuel prostate cancer cells. However, ADT can lead to adverse effects including high cholesterol, fatigue, bone loss, heart disease, anaemia, etc. Last but not least, chemotherapy uses drugs to kill or slow the growth of cancer cells. Docetaxel is a standard first-line therapy for castration-resistant prostate cancer. It is an antimicrotubular agent that binds to β-tubulin, inhibiting microtubule depolymerization, suppressing cell division, and triggering cell death. Research is also being conducted on traditional medicine, nanotechnology, and gene therapy to improve prostate cancer treatment, overcome drug resistance, and reduce side effects. Methodology Throughout my research, I gathered my sources from authoritative databases including PubMed by the National Institution of Health, Elsevier ScienceDirect, and influential textbooks from world-leading universities including Molecular Biology of the Cell and Campbell Biology. During my indexing, I used the queries of “Prostate Cancer AND Epigenetic Changes”, “Prostate Cancer AND Treatments” and “Prostate Cancer AND Treatments AND Resistance” as well as keywords including prostate cancer, DNA methylation, histone modifications, epigenetic drugs, DNMT inhibitors and HDAC inhibitors. After selecting and filtering valid and appropriate articles including literature reviews and experimental studies, I read them thoroughly and highlighted key phrases to be implemented and cited in this research essay. Only articles written in English were collected. This elicited one of the main limitations in my methodology, that is, the lack of first-hand resources in comparison to many existing experimental studies conducted by more acknowledged professionals. Another limitation of my methodology is the limited access to sources from more recent journals, conferences and confidential research. However, I still managed to integrate the experimental results from multiple studies and papers to reach my conclusion. I use the Vancouver format for my citation as it is one of the major formats used for studies including biological sciences and medicines. Epigenetic Changes in Prostate Cancer Behind lung cancer, prostate cancer is the second-leading cancer in America. According to the American Cancer Society, prostate cancer accounts for the death of 1 in 44 men. In general, histone acetylation and DNA methylation are the main epigenetic changes commonly detected in prostate cancer cells. They function either individually or collectively in the progression of cancer tumours (1). However, the reversibility of alterations in the epigenome created potential treatment methods that seek to reform the epigenome in normal cells, thereby reactivating silenced or silencing overexpressed genes. Several aberrations in the prostate epigenome include DNA hypermethylation, hypomethylation, and histone acetylation. To begin with, DNA hypermethylation is one of the most commonly investigated epigenetic aberrations in cancer. Dating back to as far as 2005 Li LC et al. obtained 52 articles reporting over 30 hypermethylated genes in prostate cancer (1). Within hypermethylated genes, silencing of cell-cycle control genes, DNA damage repair genes and hormone response genes are established important roles in prostate cancer development. Cancer tumours are often the result of the unregulated proliferation of cancer cells, in which the period of the cell cycle is reduced to increase the rate of cell division. Conventionally, checkpoints are located at the end of every phase to ensure the functionality of the cells by arresting the cell cycle when abnormalities such as DNA damage are presented. The cell checkpoints are regulated by intricate modulation systems, one of the modulators in retinoblastoma (RB1) protein (38). Thus, silencing of cell-cycle control genes by hypermethylation enables malfunctioning cancer cells to skip checkpoints that ensure their integrity, which can be achieved by tumour suppressor genes. Their product arrests the cell cycle when severe DNA damage occurs. The initial tumour suppressor gene to be discovered was RB1, and alterations in this gene have been detected in numerous types of tumours, including prostate cancer (2). However, the reason behind RB1 inactivation is ultimately found to be a result of mutation instead of hypermethylation (3)(39). Another type of tumour suppressor gene are CDKIs which obstruct the binding of RNA polymerase to the promoter region, thereby repressing transcription. Changes in CDKI expression are also reported to be responsible for cell cycle arrest (4, 5), and methylation-induced inactivation of the CDKN2A gene has been documented in prostate cancer cell lines and tissues (6)(40). DNA repair is a crucial mechanism that ensures the completeness and integrity of the cell genome during replication. Aberrations in DNA damage repair genes may lead to a higher rate of faulty mutation which leads to cell proliferation. Hypermethylation on two DNA repair genes have been observed in prostate cancer cells: GSTP1, a detoxifier gene and MGMT, another DNA repair gene. GSTP1 is a gene responsible for carcinogen detoxification (7). In prostate cancer, methylation of the GSTP1 gene promoter is the most commonly observed epigenetic change, occurring in a frequency from 70% to 100% of prostate cancer DNA samples (8-10). MGMT reduces alkyl adducts from the genome, it also repairs DNA thereby preventing point mutations. Tumours with deficient MGMT expression are more prone to point mutations in the genes that encode p53 and K-ras, potentially impacting the progression of cancer. Unlike hypermethylation which silences genes, hypomethylation involves the demethylation of conventionally methylated genes, leading to an overexpression of some genes. Hypomethylation could be separated into two major groups, those are global and gene-specific hypomethylation. Global hypomethylation represents a general decrease in methylation levels across the entire genome of a cell or tissue. In 1987, Bedford et al. found that the methylation content in DNA from metastatic tumours was significantly lower compared to nonmetastatic prostate tumours. Gene-specific hypomethylation, however, represents a reduction in DNA methylation levels at specific gene loci or promoters, rather than affecting the entire genome. In prostate cancer, the PLAU gene, which encodes a tumour-promoting protein and is conventionally methylated, is found to be heavily expressed and promotes cell proliferation (11). Histone modifications such as histone acetylation are also important regulators of gene expression in the epigenome. Coxsackie and adenovirus receptor (CAR) is a receptor for adenoviruses and their identification. Reduced expression of the CAR gene has been observed in prostate cancer and is correlated with higher Gleason scores (12). In urogenital cancer cells, such as the PC-3 prostate cancer cell line, histone acetylation regulates the activation of the CAR gene, which can be stimulated by depsipeptide, an inhibitor of histone deacetylases (HDACs)(13). Histone methylations, though rare, are also present in prostate cancer. In the prostate cancer cell line LNCaP, methylation of H3 at lysine 4 is linked to the silencing of the prostate-specific antigen (PSA) gene. Additionally, transcription of the PSA gene mediated by the androgen receptor (AR) is associated with a rapid reduction in di- and trimethylated H3 at lysine 4(41). Apart from functioning separately, sufficient evidence has reported that DNA methylation and histone modifications work together to decrease gene expression, by establishing a chromosome that is inactive in transcription as a result of binding to methylated DNA binding proteins like MeCP2. This binding subsequently attracts HDAC activity to methylated promoters, leading to the decrease in gene expression. In prostate cancer cells, DAB2IP expression is activated by the interaction between 5-aza-deoxycytidine, a demethylating agent, and trichostatin A (TSA), an HDAC inhibitor (42). Furthermore, DNA methylation and histone methylation may also cooperate to regulate gene expression. In LNCaP cancer cells, the silencing of the GSTP1 gene involves a series of sequential changes, starting with DNA methylation, followed by histone deacetylation and then histone methylation (14, 15). Epigenetic Treatments for Prostate Cancer Epigenetic modifications are best known for their specificity and reversibility. Throughout decades, their specificity has provided effective prostate cancer detection and classification (1), and their reversibility has offered many solutions that involve the mechanism of targeting potential biomarkers to up or down-regulate gene expression. The core of epigenetic treatments serves to reverse the abnormal modifications in the genome detected in prostate cancer cells, namely DNA hypermethylation, hypomethylation, and histone acetylation so that their function can be retained. To begin with, recovering the original epigenome from hypermethylation may require one of two methods, either inhibiting DNA methyltransferases or exposing the epigenome to known demethylating agents. 5-Azacytidine and 5-aza-deoxycytidine, or decitabine, serve as an inhibitor of DNA methyltransferases and it is one of the earliest inhibitors discovered. In 2014, Naldi et al. developed a brand-new drug-delivering pathway, by encapsulating decitabine in EMHVs (elastin-like polypeptide-modified hyaluronic acid nanoparticles) and further demonstrated a remarkable reduction in tumour mass in prostate cancer xenograft models (16). Furthermore, similar effects occurred on one of the highly aggressive forms of prostate cancer named neuroendocrine prostate cancer (NEPC), in which DNA methyltransferases (DNMTs) are found at elevated levels as a result of DNA hypermethylation and are strongly associated with tumour progression and metastasis. In a study conducted in November 2023, Yamada and colleagues investigated the effects of decitabine in NEPC using mouse models. They confirmed that treatment with decitabine showed promise in reducing tumour growth in both NEPC and RB1-deficient castration-resistant prostate adenocarcinoma (CRPC) models corresponding to their control group, that mice with DNMT genes knocked down also experienced reduced tumour development and metastasis. In addition, the study also introduced that decitabine treatment may lead to an increase in the expression of B7 homolog 3 (B7-H3), a protein that is typically expressed at low levels in prostate cancer cells. The researchers combined decitabine treatment with DS-7300a, an antibody-drug conjugate designed to specifically target B7-H3 and demonstrated a potential new treatment strategy for prostate cancer tumours (17). Apart from recovering from hypermethylation, DNA could also be methylated to reverse hypomethylation. Prior research pointed out that a novel type of short nucleotide called methylated sense oligonucleotide can alter DNA methylation in a sequence-specific manner both in lab and natural conditions. This method involves a synthetic oligonucleotide that has 5-methylcytosine instead of cytosine. When the methylated sense oligonucleotide binds to one DNA strand, it creates a hemi-methylated DNA intermediate that is favoured by DNMTs that further methylate the other strand and extend the methylation around the target site. Using this technique, a methylated sense oligonucleotide that targets the promoter of the ESR2 gene was introduced into PC-3 prostate cancer cells, leading to site-specific methylation and silencing of ESR2 gene expression, which is upregulated in metastatic prostate cancer due to a demethylated promoter (18). In addition, some treatments also involved the recovery of the cell from histone acetylation. This may be achieved by either inhibiting histone deacetylase activity or exposing the epigenome to known acetylating agents such as histone acetyltransferases. Histone deacetylase inhibitors block the activity of HDAC to prevent potential deacetylation, thereby increasing gene expression. Histone deacetylases (HDACs) are crucial in driving prostate cancer progression. They are involved in a transcriptional co-repressor complex that impacts several tumour suppressor genes. Given their pivotal role in cancer, HDAC inhibitors are emerging as a promising class of chemotherapeutic agents. These inhibitors have demonstrated the ability to halt cell growth, promote differentiation, and induce apoptosis in prostate cancer cells (19). Sato et al. investigated the impact of the HDAC inhibitor OBP-801 on AR expression and tumour cell growth in prostate cancers. They found that OBP-801 treatment effectively inhibited the growth of three prostate cancer cell lines (22Rv1, VCaP, and LNCaP) and reduced AR expression by posttranscriptional regulation, irrespective of their hormone sensitivity. In a rat model of AR-dependent prostate tumorigenesis, treatment with OBP-801 significantly increased miR-320a expression, which was high in normal human prostate luminal cells but low in prostate cancer cells, and suppressed prostate tumorigenesis (20). Most HDAC inhibitors serve to increase gene expression and they encourage the expression of genes that suppress tumours and arrest the cell cycle. Trichostatin A (TSA), sodium butyrate (21), and valproic acid are among some of those (22). For instance, sodium butyrate and TSA enhance the inhibitory effect of 1,25-(OH)2-vitamin D3 on prostate cancer tissue growth by initiating programmed cell death (23), and valproic acid causes prostate cancer cell apoptosis by upregulating several genes that promote cell death (22). However, other HDAC inhibitors include depsipeptides such as romidepsins which decrease transcription. Depsipeptides reduce PC-3 cell growth by downregulating VEGF mRNA expression even though they cause acetylated histones to accumulate in the chromatin near the VEGF gene promoter (24). Romidepsin, by blocking HDAC1 and HDAC2, can trigger apoptosis, cell cycle arrest, and DNA damage in prostate cancer cells (25). It can also prevent tumour growth and metastasis in prostate cancer animal models. Vorinostat is another small molecule inhibitor that belongs to dicarboxylic acid diamide and targets both class I and II histone deacetylases (HDACs). Studies have demonstrated its efficacy in inhibiting the growth of human prostate cancer cell lines, including PC-3, DU-145, and LNCaP. Additionally, vorinostat has been shown to suppress the growth of PC-3 xenograft tumours in preclinical models (23). To supplement, the thesis of epigenetic modifications in cancer treatments lies not only in reversing aberration observed in cancerous cells but also in coping readily with other existing cancer treatments such as radio and chemotherapy. For example, one of the factors that may influence the development of radioresistant traits in prostate cancer cells is the epigenetic modification of their chromatin structure and gene expression. In addition, the enhanced ability of these cells to repair DNA damage caused by ionizing radiation and the disruption of their normal cell cycle checkpoints also reduce their sensitivity to radiotherapy (26). Therefore, specific targetable epigenetic molecules may overcome and predict PCa radio resistance by targeting the locus specified to that region. In conclusion, the flexibility and reversibility of epigenetic modifications have made epigenetic treatments a promising cancer treatment method. It had already shown its potential from existing drug treatments by either reversing the epigenome or providing modifications that counteract the effect of genetic mutations on regulatory genes and promoter regions. With enhanced delivery techniques and targeting, epigenetic drugs are now prominent in medical research. Data Analysis There have been multiple clinical trials since the beginning of the decade, yet all of them were carried out on a small scale. In general, less progress has been observed with trials using DNMT inhibitors than those using HDAC inhibitors. In a phase II trial (NCT00384839) evaluating 5-azacytidine, 36 patients with prostate cancer (PCa) were enrolled. Among them, 19 patients exhibited a PSA-doubling time (DT) of less than 3 months. The median PSA-DT for all patients was extended compared to baseline, increasing from 1.5 months to 2.8 months. Out of the participants, only one patient demonstrated a notable 30% decline in PSA levels, while 14 patients exhibited only a marginal decrease in PSA levels (44). For a trial on the efficiency of Panobinostat (LBH589), docetaxel and prednisone, castration-resistant prostate cancer (CRPC) patients were administered oral Panobinostat at a dosage of 20 mg/m^2, specifically on days 15 of two-week cycle, for a total of two cycles in one arm of the study and patients in the other arm were given a combination therapy consisting of oral Panobinostat at a reduced dosage of 15 mg/m^2, along with intravenous docetaxel at a dose of 75 mg/m^2. Additionally, oral prednisone was administered twice daily at a dose of 5 mg throughout the 21-day cycle. Both arms aimed to assess the efficacy and safety profile of the respective treatment regimens in patients diagnosed with CRPC. The combination of Panobinostat with docetaxel and prednisone in CRPC patients led to notable toxicity, primarily presenting as dyspnea and neutropenia. However, despite these adverse effects, 63% of patients experienced a significant decline (>50%) in prostate-specific antigen (PSA) levels (43). In a study utilizing propensity-matched cohorts comprising 879 chemotherapy-exposed patients and 1611 chemotherapy-naïve patients, the overall survival rates at 18 and 30 months were found to be 76.3% versus 70.5% and 61.6% versus 56.0%, respectively. These findings indicate only a slight alleviation for chemotherapy-exposed patients in terms of overall survival compared to chemotherapy-naïve patients (28). In another chemotherapy phase II trial in 2021, 100 patients underwent cabazitaxel at a dosage of 20 mg/m2 every three weeks for up to ten cycles. The primary endpoint was the rate of PSA response. Among the participants, 65 out of 99 (66%) experienced a reduction in PSA levels of 50% or more from baseline (29). As a result, the effect of epigenetic treatments seems to already reach similar effects in comparison to chemotherapies, despite a small scale of clinical trials, thereby illustrating its potential as a rising treatment scheme. Advantage and Limitations Epigenetic treatments are a novel approach that focuses on reversing the epigenome of the patients to reverse the effect brought up by cancerous mutations. In general, the advantages and potential of epigenetic treatments include their reversibility, precision, and increased sensibility by synergistic effects. To begin with, the reversibility of epigenetic modifications allows a complete reversal of epigenetic aberrations to transform cells back to their normal expression level. From one perspective, the reversibility simplifies the approaches to cancer treatments, that is, releasing corresponding medications that serve to reverse epigenetic aberrations at certain biomarkers. Meanwhile, reversibility also increases the fault-tolerance level for treatments. For instance, enzymes and inhibitors could be applied to correct the changes even if off-target effects are observed since the base sequence of the genome is not altered. In addition, epigenetic treatments bear a higher precision in comparison to other prevalent methods. Before epigenetic treatments, chemotherapy was one of the most widely applied cancer treatments. Its main mechanism involves damaging the genetic material leading to either unsuccessful splitting or incomplete replication, thereby killing actively dividing cells. Thus, it may severely affect tissues with actively dividing cells, including hair, bone marrow, and epithelial cells during the treatment. Epigenetic treatments, on the other hand, target cancer biomarkers such as the GSTP1 gene which is specific to cancerous cells, thereby avoiding targeting other civilian cells which leads to side effects. Although many first and second generators epigenetic therapies in the last century targeted the epigenome globally which severely reduced the specificity of treatments as the drugs would often have wide impacts on many genes and lead to off-target effects, recent studies were able to integrate the dCas protein from the CRISPR-Cas9 technology as a novel drug delivering pathway. This was first proposed by Gilbert et al. in 2013 when they implemented non-coding guide RNAs to help the binding of deactivated Cas protein with the target promotors or repressors corresponding to the guide RNA sequence (30). Furthermore, epigenetic treatments can increase the effectiveness of other treatments by their synergistic effects in increasing the sensitivity of cancerous cells to certain treatments such as chemo and radiotherapy. For instance, androgen deprivation and androgen-receptor-targeting therapies are two gold-standard treatments for metastatic prostate cancer. However, acquired resistance to these treatments is a crucial challenge. In a 2022 review by Bagheri et al., researchers stressed that combinatorial therapy by epigenetic treatments that decrease protein kinase expression is a promising approach for metastatic PC treatment to decrease the tissue’s resistance to existing therapies. Later in a recent 2023 study, Murphy et al. applied anti-PD1 and anti-CTLA4 antibodies synergistically with vorinostat, a HADC inhibitor to reduce the cancerous tissue’s resistance for immune checkpoint blockade therapy, a common treatment for advanced prostate cancer (31). Despite its known advantages and potential, there are many problems and limitations due to the novelty of epigenetic treatments, including off-target effects, lack of biomarkers, resistance and the lack of valid trials. For many first and second-generation epigenetic therapies in the last century, treatments such as medicines that serve as activators or inhibitors often execute their functions globally, generating unintended changes on other gene locations, instead of specific loci. To be more specific, a DNA methyltransferase intended to limit the expression of an oncogene may alter some promoter sequences of a tumour suppressor gene adjacent to the target locus. This, unfortunately, causes a severe effect which would deteriorate the situation. Even as they avoided altering some important regulatory sequences in the cell cycle, they are still likely to interfere with the normal growth and function of the cell if not guided by a probe. Meanwhile, achieving high specificity for the targeted genes remains a great challenge, and there has been intense research ongoing focusing on understanding the mechanisms underlying off-target effects to decrease their off-target effects. Since the human prostate is a major endocrine gland that secretes androgen and semen in men, prostate cancers are often treated with hormonal therapies. During the first few years of clinical trials and applications, the treatment seems to work effectively. However, scientists found that resistance eventually developed in cancerous cells, leading to castration-resistant prostate cancer (CRPC). MicroRNAs (miRNAs) are a group of tiny RNA molecules that do not code for proteins. They control gene expression by either preventing the translation of target messenger RNAs (mRNAs) into proteins or by promoting the degradation of these target mRNAs (32). They are found to be dysregulated in cancerous cells resistant to bicalutamide, a common hormonal therapy drug (33). Normally, miRNAs act as transcriptional regulators by attaching to specific sequences in the 3’-untranslated region (3’-UTR) of target mRNAs (34). When miRNAs are not functioning correctly, it can disrupt various cellular and biological processes. This disruption can play a role in the development and advancement of human cancers by affecting different aspects of tumour biology, such as cell proliferation (35). Although researchers have studied many potential biomarkers in human prostate cancer, the mechanism of the interactions between the gene sequences in cancer cells is still undetermined. For instance, there is substantial evidence supporting the potential of DNA methylation detection as a valuable tumour biomarker. However, in the context of prostate cancer, only the GSTP1 gene demonstrates the necessary specificity and sensitivity for further evaluation as a tumour marker in body fluids. Even if new targets and biomarkers are proposed, there are not sufficient trials and validation for efficacy and precision due to the lack of research and some ethical issues, which is a major disadvantage for epigenetic treatments (26). Conclusion Some successes of this research are that I successfully explained the mechanism and logic of the approach, integrated recent findings in a cutting-edge area, included practical applications of known epi-drugs, and compared the effectiveness of epigenetic treatments with alternatives. To be more specific, I gathered clinical trial data for standard chemotherapy as a comparison to epigenetic treatments. Since I am not an expert in the field, the resource gathering cost me a lot of time. However, the limited clinical trials are still able to provide crucial and positive insights into the effectiveness of the treatment in comparison to chemotherapy. There were indeed some slight regrets to this research. To begin with, no first-hand resources and data are generated during my research period. This from one perspective limited the impact of this research essay. In addition, I underestimated the content of my field of research so I planned a bit over the word limit, so I did not introduce all prevalent treatments and drugs responsible. Finally, since epigenetic treatment for cancer is still a novel research field with only a few clinical trials carried out in the last decade, the impact of the trials was limited and the results were often incomprehensive to distinguish whether epigenetic treatments are more effective than chemotherapy. In a nutshell, the thesis of epigenetic treatments is derived mainly from the reversibility of epigenetic modifications and their aberrations detected in prostate cancer tissues. Naturally, epigenetic modifications are written in the chromosome by enzymes such as DNMTs and HDACs. Current epigenetic drugs mainly serve as either analogs or inhibitors for those enzymes to alter the epigenome. In this research essay, I summarised several aberrations observed in prostate cancer cells and explained some of the most often used first and second-generation drugs as well as their interactions with the transcriptional landscape and chromatin structure of our genes. Due to the novel research field, there remain some drawbacks of treatment due to our deficiency of knowledge in the underlying interactions between some enzymes, inhibitors and the epigenome. As one of the most commonly discussed and studied areas in molecular biology, epigenetics and their mechanism of interaction with the genome remained unclear for scientists. We still have a lot to figure out, such as the mechanism behind selective methylation, and the coexistence of hypermethylation and hypomethylation in prostate cancer cells before we can apply epigenetic drugs more efficiently. Although results from small clinical trials in the last decade remain fluctuating, some have already provided promising results that, in some circumstances, outweigh chemotherapeutics. Moreover, ongoing clinical trials have been continuing to provide valuable insights to the field which is making this treatment a feasible and viable approach to prostate cancer. Thus, there is still a bright future ahead for the application of epigenetic engineering to prostate cancer treatments, while trials must be carried out with caution until we have a clear understanding of the mechanisms underlying epigenetic interactions. Bibliography and References 1.Li LC, Carroll PR, Dahiya R. Epigenetic changes in prostate cancer: implication for diagnosis and treatment. J Natl Cancer Inst. 2005;97(2):103-15. 2.Lee WH, Bookstein R, Hong F, Young LJ, Shew JY, Lee EY. Human retinoblastoma susceptibility gene: cloning, identification, and sequence. Science. 1987;235(4794):1394-9. 3.Kubota Y, Fujinami K, Uemura H, Dobashi Y, Miyamoto H, Iwasaki Y, et al. Retinoblastoma gene mutations in primary human prostate cancer. Prostate. 1995;27(6):314-20. 4.Aaltomaa S, Lipponen P, Eskelinen M, Ala-Opas M, Kosma VM. Prognostic value and expression of p21(waf1/cip1) protein in prostate cancer. Prostate. 1999;39(1):8-15. 5.Cote RJ, Shi Y, Groshen S, Feng AC, Cordon-Cardo C, Skinner D, et al. Association of p27Kip1 levels with recurrence and survival in patients with stage C prostate carcinoma. J Natl Cancer Inst. 1998;90(12):916-20. 6.Jarrard DF, Bova GS, Ewing CM, Pin SS, Nguyen SH, Baylin SB, et al. Deletional, mutational, and methylation analyses of CDKN2 (p16/MTS1) in primary and metastatic prostate cancer. Genes Chromosomes Cancer. 1997;19(2):90-6. 7.Nelson CP, Kidd LC, Sauvageot J, Isaacs WB, De Marzo AM, Groopman JD, et al. Protection against 2-hydroxyamino-1-methyl-6-phenylimidazo[4,5-b]pyridine cytotoxicity and DNA adduct formation in human prostate by glutathione S-transferase P1. Cancer Res. 2001;61(1):103-9. 8.Santourlidis S, Florl A, Ackermann R, Wirtz HC, Schulz WA. High frequency of alterations in DNA methylation in adenocarcinoma of the prostate. Prostate. 1999;39(3):166-74. 9.Lee WH, Isaacs WB, Bova GS, Nelson WG. CG island methylation changes near the GSTP1 gene in prostatic carcinoma cells detected using the polymerase chain reaction: a new prostate cancer biomarker. Cancer Epidemiol Biomarkers Prev. 1997;6(6):443-50. 10.Lee WH, Morton RA, Epstein JI, Brooks JD, Campbell PA, Bova GS, et al. Cytidine methylation of regulatory sequences near the pi-class glutathione S-transferase gene accompanies human prostatic carcinogenesis. Proc Natl Acad Sci U S A. 1994;91(24):11733-7. 11.Van Veldhuizen PJ, Sadasivan R, Cherian R, Wyatt A. Urokinase-type plasminogen activator expression in human prostate carcinomas. Am J Med Sci. 1996;312(1):8-11. 12.Rauen KA, Sudilovsky D, Le JL, Chew KL, Hann B, Weinberg V, et al. Expression of the coxsackie adenovirus receptor in normal prostate and in primary and metastatic prostate carcinoma: potential relevance to gene therapy. Cancer Res. 2002;62(13):3812-8. 13.Pong RC, Lai YJ, Chen H, Okegawa T, Frenkel E, Sagalowsky A, et al. Epigenetic regulation of coxsackie and adenovirus receptor (CAR) gene promoter in urogenital cancer cells. Cancer Res. 2003;63(24):8680-6. 14.Lu Q, Kaplan M, Ray D, Ray D, Zacharek S, Gutsch D, et al. Demethylation of ITGAL (CD11a) regulatory sequences in systemic lupus erythematosus. Arthritis Rheum. 2002;46(5):1282-91. 15.Segura-Pacheco B, Trejo-Becerril C, Perez-Cardenas E, Taja-Chayeb L, Mariscal I, Chavez A, et al. Reactivation of tumor suppressor genes by the cardiovascular drugs hydralazine and procainamide and their potential use in cancer therapy. Clin Cancer Res. 2003;9(5):1596-603. 16.Naldi I, Taranta M, Gherardini L, Pelosi G, Viglione F, Grimaldi S, et al. Novel epigenetic target therapy for prostate cancer: a preclinical study. PLoS One. 2014;9(5):e98101. 17.Yamada Y, Venkadakrishnan VB, Mizuno K, Bakht M, Ku SY, Garcia MM, et al. Targeting DNA methylation and B7-H3 in RB1-deficient and neuroendocrine prostate cancer. Sci Transl Med. 2023;15(722):eadf6732. 18.Lau KM, LaSpina M, Long J, Ho SM. Expression of estrogen receptor (ER)-alpha and ER-beta in normal and malignant prostatic epithelial cells: regulation by methylation and involvement in growth regulation. Cancer Res. 2000;60(12):3175-82. 19.Abbas A, Gupta S. The role of histone deacetylases in prostate cancer. Epigenetics. 2008;3(6):300-9. 20.Sato S, Katsushima K, Shinjo K, Hatanaka A, Ohka F, Suzuki S, et al. Histone Deacetylase Inhibition in Prostate Cancer Triggers miR-320-Mediated Suppression of the Androgen Receptor. Cancer Res. 2016;76(14):4192-204. 21.Suenaga M, Soda H, Oka M, Yamaguchi A, Nakatomi K, Shiozawa K, et al. Histone deacetylase inhibitors suppress telomerase reverse transcriptase mRNA expression in prostate cancer cells. Int J Cancer. 2002;97(5):621-5. 22.Thelen P, Schweyer S, Hemmerlein B, Wuttke W, Seseke F, Ringert RH. Expressional changes after histone deacetylase inhibition by valproic acid in LNCaP human prostate cancer cells. Int J Oncol. 2004;24(1):25-31. 23.Rashid SF, Moore JS, Walker E, Driver PM, Engel J, Edwards CE, et al. Synergistic growth inhibition of prostate cancer cells by 1 alpha,25 Dihydroxyvitamin D(3) and its 19-nor-hexafluoride analogs in combination with either sodium butyrate or trichostatin A. Oncogene. 2001;20(15):1860-72. 24.Sasakawa Y, Naoe Y, Noto T, Inoue T, Sasakawa T, Matsuo M, et al. Antitumor efficacy of FK228, a novel histone deacetylase inhibitor, depends on the effect on expression of angiogenesis factors. Biochem Pharmacol. 2003;66(6):897-906. 25.Karagiannis D, Rampias T. HDAC Inhibitors: Dissecting Mechanisms of Action to Counter Tumor Heterogeneity. Cancers (Basel). 2021;13(14). 26.Macedo-Silva C, Benedetti R, Ciardiello F, Cappabianca S, Jeronimo C, Altucci L. Epigenetic mechanisms underlying prostate cancer radioresistance. Clin Epigenetics. 2021;13(1):125. 27.Thibault A, Figg WD, Bergan RC, Lush RM, Myers CE, Tompkins A, et al. A phase II study of 5-aza-2'deoxycytidine (decitabine) in hormone independent metastatic (D2) prostate cancer. Tumori. 1998;84(1):87-9. 28.Hoeh B, Wurnschimmel C, Flammia RS, Horlemann B, Sorce G, Chierigo F, et al. Effect of Chemotherapy on Overall Survival in Contemporary Metastatic Prostate Cancer Patients. Front Oncol. 2021;11:778858. 29.Hofman MS, Emmett L, Sandhu S, Iravani A, Joshua AM, Goh JC, et al. [(177)Lu]Lu-PSMA-617 versus cabazitaxel in patients with metastatic castration-resistant prostate cancer (TheraP): a randomised, open-label, phase 2 trial. Lancet. 2021;397(10276):797-804. 30.Gilbert LA, Larson MH, Morsut L, Liu Z, Brar GA, Torres SE, et al. CRISPR-mediated modular RNA-guided regulation of transcription in eukaryotes. Cell. 2013;154(2):442-51. 31.Murphy S, Rahmy S, Gan D, Zhu Y, Manyak M, Li J, et al. Overcome Prostate Cancer Resistance to Immune Checkpoint Therapy with Ketogenic Diet-Induced Epigenetic Reprogramming. bioRxiv. 2023. 32.Zhou W, Huang S, Jiang Q, Yuan T. Suppression of miR-4735-3p in androgen receptor-expressing prostate cancer cells increases cell death during chemotherapy. Am J Transl Res. 2017;9(8):3714-22. 33.Chow H, Ghosh PM, deVere White R, Evans CP, Dall'Era MA, Yap SA, et al. A phase 2 clinical trial of everolimus plus bicalutamide for castration-resistant prostate cancer. Cancer. 2016;122(12):1897-904. 34.Li CY, Wang YH, Lin ZY, Yang LW, Gao SL, Liu T, et al. MiR-5100 targets TOB2 to drive epithelial-mesenchymal transition associated with activating smad2/3 in lung epithelial cells. Am J Transl Res. 2017;9(10):4694-706. 35.Li K, Pan J, Wang J, Liu F, Wang L. MiR-665 regulates VSMCs proliferation via targeting FGF9 and MEF2D and modulating activities of Wnt/beta-catenin signaling. Am J Transl Res. 2017;9(10):4402-14. 37. Chen H, Toyooka S, Gazdar AF, Hsieh JT. Epigenetic regulation of a novel tumor suppressor gene (hDAB2IP) in prostate cancer cell lines. J Biol Chem 2003;278:3121–30. 38. Fernandez PL, Jares P, Rey MJ, Campo E, Cardesa A. Cell cycle regu- lators and their abnormalities in breast cancer. Mol Pathol 1998;51:305–9. 39. Konishi N, Nakamura M, Kishi M, Nishimine M, Ishida E, Shimada K. Heterogeneous methylation and deletion patterns of the INK4a/ARF locus within prostate carcinomas. Am J Pathol 2002;160:1207–14. 40. Herman JG, Merlo A, Mao L, Lapidus RG, Issa JP, Davidson NE, et al. Inactivation of the CDKN2/p16/MTS1 gene is frequently associated with aberrant DNA methylation in all common human cancers. Cancer Res 1995;55:4525–30. 41. Strahl BD, Ohba R, Cook RG, Allis CD. Methylation of histone H3 at lysine 4 is highly conserved and correlates with transcriptionally active nuclei in Tetrahymena. Proc Natl Acad Sci U S A 1999;96:14967–72. 42. Chen H, Toyooka S, Gazdar AF, Hsieh JT. Epigenetic regulation of a novel tumor suppressor gene (hDAB2IP) in prostate cancer cell lines. J Biol Chem 2003;278:3121–30. 43. Rathkopf D, Wong BY, Ross RW, Anand A, Tanaka E, Woo MM, et al. A phase I study of oral Panobinostat alone and in combination with docetaxel in patients with castration-resistant prostate cancer. Cancer Chemother Pharmacol. 2010;66(1):181–9. 44. Sonpavde G, Aparicio AM, Zhan F, North B, Delaune R, Garbo LE, Rousey SR, Weinstein RE, Xiao L, Boehm KA, Asmar L, Fleming MT, Galsky MD, Berry WR, Von Hoff DD. Azacitidine favorably modulates PSA kinetics correlating with plasma DNA LINE-1 hypomethylation in men with chemonaïve castration-resistant prostate cancer. Urol Oncol. 2011;29(6):682–9. doi:10.1016/j.urolonc.2009.09.015.
Exploration and determination of lung cancer targets using multi-omics approaches
Liang Chenyu1, †, Pinyan Rosemary Duan2, 3, † 1Ulink College of Shanghai, No 559, South Laiting Road, Songjiang District, Shanghai, 201615 2 (Wellington China) Huili School Shanghai 3 rosemary.duan.2026@huilieducation.cn †All authors contributed equally. Abstract. Multi-omics analysis is a relatively new approach which sets of data are combined for analysis. It integrates the genomic, epigenomic, transcriptomic, proteomic, and metabolomic data from diseased individuals and is capable of comparing the different changes in levels of each identified molecules with the assist of charts and graphs by information technologies, ultimately proposing potential biomarkers and correlations that are possible targets for cancer drugs. Lung cancer, nowadays is the most common cancer being diagnosed worldwide. Thus, lung cancer, amongst the remaining cancer, should not be overlooked. The pathogenesis and treatment of lung cancer is an urgent problem in the medical field that needs to be researched thoroughly. By integrating this new approach to the most common cancer, we try to find more possibilities for targeted drug development. We analyzed the data from the existing database to find out whether the control group and the disease group were up-regulated or down-regulated to analyze some targets, contributing to the development of targeted drugs. Keywords: Lung adenocarcinoma, Multi-omics, Drug targets. 1. Introduction Lung cancer is the most common cancer and one of the main causes of cancer-related deaths worldwide. According to GLOBOCAN 2020, there are an estimated 2.2 million new lung cancer cases (11.4%) and nearly 1.8 million lung cancer deaths (18.0%) in 2020. China had the highest number of lung cancer cases and mortality rates (37.0% and 39.8%, respectively) in 2020, posing a serious threat to the public health [1]. Thus, it shows that lung cancer should not be overlooked and the pathogenesis and treatment of lung cancer is an urgent problem in the medical field that needs to be researched thoroughly. The occurrence of lung cancer is caused by the activation of oncogenes or the inactivation of tumor suppressor genes, which leads to uncontrolled replication and growth of lung cells. Tumors can disrupt the normal structure of lung tissue, block nutrient or oxygen supply, and affect normal lung function. The two most common causes are specific genetic mutations and environmental carcinogens. Tobacco is the most common risk factor for cancer. More than 4000 chemical substances have been found in tobacco, including at least 69 known carcinogens and other toxic substances related to serious diseases [2]. © 2024 The Authors. This is an open access article distributed under the terms of the Creative Commons Attribution License 4.0 (https://creativecommons.org/licenses/by/4.0/). 34 Proceedings of the 2nd International Conference on Modern Medicine and Global Health DOI: 10.54254/2753-8818/33/20240767 At present, the most direct treatment method for lung cancer is surgical resection of the cancer tumor. In the early stages, complete resection of lung tumors is possible. When lung cancer progresses to the advanced stage, when the tumor is too large or has already spread, it is difficult to completely remove the tumor tissue through surgery, which also limits the possibility of some patients undergoing surgery. Chemotherapy and radiation therapy are auxiliary treatment methods for cancer, which can be used to reduce tumor size before surgery. However, the side effects of chemotherapy and radiation therapy are very significant, not only causing significant physical harm to patients, but also causing harm to their mental health, seriously affecting their quality of life. Targeted therapy is a type of cancer treatment that targets proteins that control the growth, division, and spread of cancer cells. It is the foundation of precision medicine. It helps the immune system destroy cancer cells, cut off signals that help form blood vessels, and ultimately kill cancer cells. This therapy is widely used because it can accurately destroy cancer cells with relatively less obvious side effects. As long as the patient has a good target, targeted treatment will be very effective. Thus, this article aims to analyze the targets of cancer patients through multi-omics approach, to seek more possibilities to develop more effective targeted drugs to treat lung cancer. In order to shed light on the development of targeted medications to lung cancer, scientists first are required to obtain a deeper understanding of the relationships between multiple aspects and features of the lung adenocarcinoma cells so that drugs targeting the specific signal transduction pathway, protein expression or metabolites could be established. In order to obtain a more comprehensive understanding of the correlation between multiple disease-associated features and molecular levels, multi-omics analysis integrates the genomic, epigenomic, transcriptomic, proteomic, and metabolomic data from diseased individuals and is capable of comparing the different changes in levels of each identified molecules with the assist of charts and graphs by information technologies, ultimately proposing potential biomarker. Furthermore, it offers a theoretical basis for researching and developing target drugs. Various multi-omics approaches had previously been done to lung cancer treatments in recent years. Back as soon as in 2020, Asada et al. integrated RNA and miRNA expressions, compared 25 cancer related genes and filtered out 6 potential genes influencing that survival rates of patients [3] combined data of DNA methylation, RNA, miRNA, and DNA copy number and determined in total of six cancer subtypes and their corresponding survival rate related genes on the chromosome [4]. Subsequently, specific oncogene NUF2 and regulators of radiotherapy resistance such as GALNT2 are discovered in 2021, 2023 respectively [5, 6]. As a new technology integrating etiology with data science, researches on lung adenocarcinoma and its related targets remained superficial and incomprehensive. Despite its various advantages, drawbacks of multi-omics approaches remained significant. To begin with, due to the differences in size, format and resolution among multiple data sets, multi-omics data are often hard to integrate for analysis. Moreover, due to differences in experimental procedures and equipment, multi-omics data sets are often scarce and incomplete. In addition, analysis and comparation between multi-omics data sets may generate confounding results leading to undecipherable correlations which decreases the reliability of the results, this need to be carefully analyzed be experts with prevalent data sets. Finally, multi-omics data usually involves patients’ private information, which may raise concerns about its privacy protection [7]. For example, omics data may reveal genetic information, cancer variants or treatment biomarkers related to diseases or characteristics that are private to patients. Therefore, it is important to no violate any personal privacy and ethical concerns while dealing with multi-omics data sets. As a result, we integrated existing lung adenocarcinoma multi-omics data sets and analyzed the correlation in the new method, seeking to provide valuable insights to targeted drug development and clinical medications to lung adenocarcinoma. 2. Method Firstly, we downloaded our lung adenocarcinoma related multi-omics data sets from CBioPortal and extracted mRNA and RPPA related data for further analysis. We analyzed the intersection of the two data sets using Boolean logic and filtered their co-expressed targets. Then, we calculated the difference 35 Proceedings of the 2nd International Conference on Modern Medicine and Global Health DOI: 10.54254/2753-8818/33/20240767 in expression of the target genes, sorted the 10 genes with highest increased and decreased expression, respectively, and speculated treatment targets for the progression of lung adenocarcinoma. 2.1. Read By using “read_csv” method in “pandas” pack, a data file with dataframe structure is read. Input “data_rppa_zscores.txt” and “data_mrna_seq_v2_rsem_zscores_ref_all_samples.txt” to the dataframe, then use “head” methodology to read the first five lines in order to examine if all datas are read correctly. 2.2. Examination The examination of the presence of “TGCA-44-2657-01” in two dataframes is done at the start by using the following code, since the intersection of data column “TGCA-44-2657-01” need to be obtained afterwards. data_rppa_columns[1] in data_rna.columns A variable of Boolean type is returned, and the variables of this type can only be “true” or “false”. If the above code returns “true”, it means that there is a column named “TCGA-44-2657-01” in both data boxes, then the data can continue to be analyzed. Otherwise the analysis terminates 2.3. Intersection The following code is used to obtain common elements (intersections) of this data column after conforming the existence of the common column. common_data = np.intersectid(data_rppa[‘TCGA-44-2657-01’],data_rna[‘tcga-44-2657-01’]) 2.4. Filtration Moreover, only obtaining common elements are not enough, the name of genes corresponding to these common elements need to be excavated as well. Therefore, filtration is done by inputting these common elements back to the original dataframe by the following codes. rppa_filter_condition = data_rppa[‘TCGA-44-2657-01’].isin(common_data) rppa_wanted_cols = [‘ Composite. ELement. REF*, ‘TCGA-44-2657-01*] rppa_data_after_filter = data_rppa. LocLrppa_ Fil ter_condition, rppa_wanted_colsJ- sort-valuesC” TCGA- 44-2657- 011) Especially, “rppa_filter_condition = data_rppa[‘TCGA-44-2657-01’].isin(common_data)”is used as a condition for filtration. If a row of data_rppa dataframe, which is a gene sample, has its “TCGA-44 2657-01” data present in common_data, then this row will be labelled as “true”. Therefore, “rppa_filter_condition” and “data_rppa” will obtain the same Boolean type classified by the same row number. This Boolean type will be used as filter condition to filtrate the data of “data_rppa”, then the corresponding gene names are obtained. The “rna_data” dataframe is opperated like this as well. following code is used to obtain common elements (intersections) of this data column after conforming the existence of the common column. 2.5. Output From the filtering step above, we not only acquired the name of the genes from the intersection of two data groups, but also used “sort_values” to arrange them in order, and chose the only top ten and bottom ten data values using the following code: 36 Proceedings of the 2nd International Conference on Modern Medicine and Global Health DOI: 10.54254/2753-8818/33/20240767 rppa_index data_after_filter))]) =np.concatenate([np.arange(10),np.arange(len(rppa_data_after_filter)-10,len(rppa_ rppa_result = rppa_data_after_filter.iloc[rppa_index,:] In the table, “rppa_result” represented the final result for RPPA data, and it is the same method for RNA data. After we obtained the first and last ten rows from the table, what we got is still to data sets, we still need to merge the tables to become a single table. As we have a common column of “TCGA 44-2657-01”, we are able to merge two tables base on this common key using internal combination using the following code: final_ret = pd.merge(rna_result,rppa_result,on=‘TCGA-44-2657-01’) final_ret.to_csv(“merged_data.csv”) And the following code is used to rewrite the final result in a .csv file final_ret.to_csv(“merged_data.csv”). 2.6. Integration The previous five steps generates a result for only one person; however, we need to integrate more data to obtain a more accurate and comprehensive result. First, we need to identify all patients both present in the RPPA and the mRNA set but the following code. common_cols = np.intersect1d(data_rppa.columns,data_rna.columns) Then, we perform the following code to merge the RPPA and mRNA data for each patients and download their data individually to obtain a csv document, or table, for each of the 181 patients. The logic of the program is identical to what was explained previously in the five steps. def data_reshape(col,cnt): common_data = np.intersect1d(data_rppa[col],data_rna[col]) print(str(cnt),”:”,col,str(common_data.shape[0])) rppa_filter_condition = data_rppa[col].isin(common_data) rppa_wanted_cols = [‘Composite.Element.REF’,col] = rppa_data_after_filter = data_rppa.loc[rppa_filter_condition,rppa_wanted_cols].sort_values(col) rppa_index np.concatenate([np.arange(10),np.arange(len(rppa_data_after_filter) 10,len(rppa_data_after_filter))]) rppa_result = rppa_data_after_filter.iloc[rppa_index,:] rna_filter_condition = data_rna[col].isin(common_data) rna_wanted_cols = [‘Hugo_Symbol’,’Entrez_Gene_Id’,col] = rna_data_after_filter = data_rna.loc[rna_filter_condition,rna_wanted_cols].sort_values(col) rna_index np.concatenate([np.arange(10),np.arange(len(rna_data_after_filter) 10,len(rna_data_after_filter))]) rna_result = rna_data_after_filter.iloc[rna_index,:] final_ret = pd.merge(rna_result,rppa_result,on=col) final_ret.to_csv(col+”.csv”) print(col+’.csv’+’ download’) 37 Proceedings of the 2nd International Conference on Modern Medicine and Global Health DOI: 10.54254/2753-8818/33/20240767 for i,col in enumerate(common_cols): data_reshape(col,i+1) We define a function “data_reshape” that takes two arguments: “col” and “cnt”. The parameter “col” is a string indicating the name of the column to be processed, and “cnt” is an integer indicating the position of the currently processed column in the common_cols list. The main operation of the function is as follows. First, we find the intersection of the two datasets with the “np.intersectld” function and store the result in the “common_data” variable. Then we printed the names of the columns currently being processed, the number of intersections, and the names of the columns. Next, we created some variables for storing the filtered RPPA and RNA data and the names of the columns to be retained. After, we filtered the RPPA data that satisfies the condition by “data_rppa[col].isin(common_data)” and stored the result in the rppa_filter_condition variable; we selected the desired columns from data_rppa based on the filter condition and stored the result in the “rppa_wanted_cols” variable. Later, we sorted the filtered RPPA data, stored the result in the “rppa_data_after_filter” variable, calculated the index to be retained and stored the result in the “rppa_index” variable. Finally, we selected data from the “rppa_data_after_filter” based on the index and stored the result in the rppa_result variable. Similarly, we filtered, sorted, and performed selected operations on the RNA data and the results are stored in the rna_result variable. And now we have acquired the data from both data and we need to combine them effectively to show the results more intuitively. We used the “pd.merge” function to merge the RPPA and RNA data, stored the results in the final_ret variable, saved the merged data as a CSV file and printed out the file name. Finally, the code iterates through each column in the common_cols list with a loop and passes each column to the data_reshape function for processing. The code above generated, in total, 181 csv documents for us, and we are going to carry out four procedures to visualize our data: arranging the z-scores for each patient, filtering out the 10 highest and lowest z-score values, counting which gene is most appeared for the all patients, and finally calculating the z-score average. os.chdir(‘./CSV’) min_list = np.array([]) max_list = np.array([]) for f in os.listdir(): if not f.startswith(‘.’): csv = pd.read_csv(f) min_list = np.concatenate([min_list,csv[‘Hugo_Symbol’][:10].values]) max_list = np.concatenate([max_list,csv[‘Hugo_Symbol’][10:].values]) majority_min = pd.Series(min_list).value_counts()[:10] majority_min majority_max = pd.Series(max_list).value_counts()[:10] majority_max This code gets all the CSV files in the current directory and reads them as DataFrame objects. Then, for each CSV file, it extracts the first 10 values and the last 10 values of the gene symbol (Hugo_Symbol) column in it and adds them to the min_list and max_list arrays respectively. Specifically, the code first creates two empty arrays “min_list” and “max_list”, and then uses the “os.listdir()” function to get all the files in the current directory. For each file, if it does not start with “.” it means that it is a CSV file and can be read as a DataFrame object using “pd.read_csv(f)”. 38 Next, the code uses “csv[‘Hugo_Symbol’][:10].values” to extract the first 10 values of the gene symbol column in each CSV file and adds them to the min_list array using the “np.concatenate()” function. Similarly, the code uses “csv[ ‘Hugo_Symbol’][10:].values” to extract the last 10 values of the gene symbol column in each CSU file and adds them to the max_list array using the “np.concatenate()” function. Finally, the code prints out the min_list and max_list arrays separately in order to view their contents. Table1. Most appeared genes with minimum z scores Hugo_Symbol Appearances CASP14 4 GMPPA 3 SLC1A5 3 LMOD2 3 ATCAY 3 BARHL2 3 KLK15 3 TEX13A 3 IL20RB 3 NXF5 3 Table 2. Most appeared genes with minimum z scores Hugo_Symbol Appearances RPL37 3 NT5E 3 NPRL3 3 ZNF10 3 PAPOLG 2 WDR77 2 HIF1AN 2 UQCRFS1 2 DNTTIP1 2 IFNAR2 2 We then calculated the average z-score values for the expression of the genes above using the following code. min_mean_list = [] for f in os.listdir(): if not f.startswith(‘.’): csv = pd.read_csv(f) csv = csv.iloc[:10,:] temp_mean_list = [] for col in majority_min.index: if col in csv[‘Hugo_Symbol’].values: Proceedings of the 2nd International Conference on Modern Medicine and Global Health DOI: 10.54254/2753-8818/33/20240767 39 Proceedings of the 2nd International Conference on Modern Medicine and Global Health DOI: 10.54254/2753-8818/33/20240767 # temp_df = csv[csv[‘Hugo_Symbol’] == col] print(temp_df) mean_value = temp_df[f.split(‘.’)[0]].mean() temp_mean_list.append(mean_value) else: temp_mean_list.append(0) min_mean_list.append(temp_mean_list) min_mean_arr = np.array(min_mean_list) min_ret = min_mean_arr.mean(axis=0) We then carried out the same procedures for genes that appeared the most in the table. max_mean_list = [] for f in os.listdir(): if not f.startswith(‘.’): csv = pd.read_csv(f) csv = csv.iloc[10:,:] temp_mean_list = [] for col in majority_max.index: if col in csv[‘Hugo_Symbol’].values: temp_df = csv[csv[‘Hugo_Symbol’] == col] # print(temp_df) mean_value = temp_df[f.split(‘.’)[0]].mean() temp_mean_list.append(mean_value) else: temp_mean_list.append(0) max_mean_list.append(temp_mean_list) max_mean_arr = np.array(max_mean_list) max_ret = max_mean_arr.mean(axis=0) min_df = pd.DataFrame({‘Hugo_Symbol’:majority_min.index,’value’:min_ret}) min_df.to_csv(‘min_ret.csv’) max_df = pd.DataFrame({‘Hugo_Symbol’:majority_max.index,’value’:max_ret}) max_df.to_csv(‘max_ret.csv’) The main purpose of this code is to read all the CSV files in the current directory and calculate the average value of each gene for each file. The code first creates two lists, “min_mean_list” and “temp_mean_list”, and then uses the “os.listdir()” function to get all the files in the current directory. For each file, if it does not start with “.” it means that it is a CSV file, and you can use “pd.read_csv(f)” to read it as a DataFrame object. 40 Proceedings of the 2nd International Conference on Modern Medicine and Global Health DOI: 10.54254/2753-8818/33/20240767 Next, the code uses “csv.iloc[:10,:]” to extract the first 10 rows of data from each CSV file and stores the result in a CSV variable. The code then creates an empty “temp_mean_list” list for storing the mean values for each gene. For each element in the “majority_min” index (i.e., each gene), perform the following. If the gene is in the Hugo_Symbo1 column of the CSV, extract the row containing the gene from the CSV and store the result in the temp_df variable. Use “temp_df[f.split(‘.’)[0]].mean()” to calculate the mean value for that gene in that file and add the result to “temp_mean_list”. If the gene is not in the “Hugo_Symbo1” column of the CSV, add 0 to “temp_mean_list”. Finally, the code adds the temp_mean_list to the “min_mean_list” and prints out “min_mean_list” to see its contents. We then carried out the same procedures for genes that appeared the most in the table, and the result of the final table is presented in the results section as Fig. 3. Result To begin our analysis, we downloaded our multi-omics data sets of patients diagnosed with lung adenocarcinoma from CBioPortal by searching “tcga luad”, in which TCGA stands for “The Cancer Genome Atlas Program” and LUAD stands for “Lung Adenocarcinoma”. Then, we selected mRNA and RPPA related data for further analysis using python. Firstly, we modelled the expression of mRNA strands and proteins in two normal distribution, and calculated z-scores for the expression levels for each gene in each patient. As there is a direct link between transcription and translation, if a mRNA strand is synthesised, the corresponding protein is also very likely to be synthesized. Furthermore, mRNA and protein expression levels with the same z-score after standardisation also represent a likely correlation their probability of expression are the same. As a result, we analysed the intersection of the two data sets using Boolean logic to find, if any, genes of which mRNA and protein expression converges. Then, we ranked the difference in z-score of the target genes and sorted the 10 genes with highest increased and decreased expression, respectively, and speculated treatment targets for the progression of lung adenocarcinoma. The flow chart of the analysis is listed below. Figure 1. Multi-omics analysis flow chart. The results of the analysis are listed as follow. 41 Figure 2. Intersection results of proteome and transcriptome. In total, 81 genes were found to exist having the same z-score level of mRNA and protein expression, meaning that there is a significant correlation between each of the paired groups as they have same probability of existence in the human body. After the combination of results, we are able to filtered out the other irrelevant genes, and rank the target genes as follow according to their z-score value as follow. Table 3. List of differentially expressed genes. Hugo_Symbol Entrez_Gene_Id TCGA-44-2657-01 20422 ZP4 57829 -1.3531 4818 DEFB4A 1673 -1.2587 8567 IRS2 8660 -0.9179 11110 MRPL33 9553 -0.7430 13083 PDE12 201626 -0.6891 725 SOWAHC 65124 -0.6717 4461 CXCL5 6374 -0.6639 1115 ASPG 374569 -0.6428 6542 FMR1NB 158521 -0.6401 3688 CHMP2A 27243 -0.5369 5334 ECM2 1842 0.8386 12884 PARD6A 50855 0.8798 2568 ADGB 79747 0.8917 531 ALDH1L1 10840 0.9030 11812 NHSL2 340527 0.9191 12861 PAPD7 11044 0.9876 16152 SLC25A33 84275 0.9881 17599 TARP 445347 1.0511 20332 ZNF767 79970 1.3384 13948 PRCD 768206 1.9639 The z-score value of the expression in each protein in the patient above indicates the probability of gene expression. The z-score value is calculated by the observed value of expression deducting the mean expression value then divided by the standard deviation of the value when the data of gene expression is modeled into a normal distribution. In order to obtain more knowledge about the targets, we first searched and filtered existing targets suggested by other experts in the area via PubMed and found that IRS2 was suggested in 2022 by H. Proceedings of the 2nd International Conference on Modern Medicine and Global Health DOI: 10.54254/2753-8818/33/20240767 42 Peng et al as a direct symbol of tumorigenesis. The analysis, however, is nowhere near complete. We then calculated the average of each z-score value according to the name of the gene for over 200 patients and generalized a table of genes each with the most increased and decreased expression, and the table is listed below. Table 4. Highest 10 average z-scores of the 200 patients Rank Hugo_Symbol Value 1 RPL37 0.0202232044198895 2 NT5E 0.01805414364640884 3 NPRL3 0.018520994475138122 4 ZNF10 0.016159668508287293 5 PAPOLG 0.013338674033149171 6 WDR77 0.012006629834254144 7 HIF1AN 0.007766850828729283 8 UQCRFS1 0.013050276243093921 9 DNTTIP1 0.015307734806629836 10 IFNAR2 0.009991160220994475 Table 5. Lowest 10 average z-scores of the 200 patients Rank Hugo_Symbol Value 1 CASP14 -0.025752486187845303 2 GMPPA -0.017875138121546962 3 SLC1A5 -0.016581215469613258 4 LMOD2 -0.017814364640883977 5 ATCAY -0.018302209944751385 6 BARHL2 -0.01981160220994475 7 KLK15 -0.02165966850828729 8 TEX13A -0.01472817679558011 9 IL20RB -0.013792817679558011 10 NXF5 -0.018898342541436467 In a detailed search and examination of the functions of the 20 genes, we found 3 genes in total directly contributing to lung adenocarcinoma. Beginning with RPL37 which is ranked number 1 in the expression table above. The next genes is WDR77, ranking number 6 among the most expressed proteins, and it is found to proliferate the cells by letting then to reenter the cell cycle, ultimately forming cancer. Last but not least came IL20RB, which promotes bone metastasis of lung cancer. RPL37 RPL37 is a gene that is the source of expression of a ribosomal protein located at the 60S subunit in the cytoplasm. The L37E ribosomal proteins is the family of which the gene belongs. In a nutshell, Ribosomes catalyze the building and synthesis of proteins, they contain two subunits in total - a smaller 40S subunit and a larger 60S subunit - consist of 4 RNA strands and almost 80 structurally unique proteins. The RPL37 gene is associated with several diseases such as cataract 4, multiple types, and atrial septal defect. L. Daftuar et al. found in an 2013 article that RPL37 is among the RPs that activates p53, a tumor-suppressor gene by the downregulation of Mdm level [8]. WDR77 WDR77 is one of the WD40-containing proteins that are expressed for rapid growth of the epithelial cells for which it enables cells to join the cell cycle. Z. Gu .et al in 2013 published that the WDR77 gene Proceedings of the 2nd International Conference on Modern Medicine and Global Health DOI: 10.54254/2753-8818/33/20240767 43 Proceedings of the 2nd International Conference on Modern Medicine and Global Health DOI: 10.54254/2753-8818/33/20240767 is re-activated in cells found to be in lung adenocarcinoma and caused abnormally high proliferation by letting cells to re-enter the cell cycle [9]. IL-20RB IL-20RB, also known as the beta subunit of human interleukin-20 receptor, is a recombinant protein product primarily used in immunohistochemical testing. IL-20RB is mainly expressed in skin tissue and is secreted by peripheral blood mononuclear cells (PBMCs) and activated epithelial cells. Its receptor is formed by combining IL-20Ralpha and IL-20Rbeta subunits. IL-20 must bind to both IL-20Ralpha and IL-20Rbeta simultaneously to activate STAT3 transcription factor and subsequently activate the expression of downstream genes. A recent article in 2022 published by Y. He et al. suggested that IL 20RB directly promoted the metastasis of lung adenocarcinoma to the bone marrow, and proved that the reduced expression of this gene could effectively decrease the metastasis of lung adenocarcinoma cells to the bone marrow [10]. 4. Discussion Although our method of simple multi-omics analysis and integration suggested some of the possible targets related to lung adenocarcinoma, there are still limitations for this experiment. In terms of data, the recorded data sets are identified through CBioPortal by searching “tcga luad”. Therefore, the experimental autonomy is relatively low since we didn’t collect the data by ourselves. In addtion, the accuracy of this dataset cannot be monitored, this leads to a problem since the data collecting process is sophisticated. Firstly, the researchers need to collect lung tissue from a lung adenocarcinoma patient (disease group), and the lung tissue from health person (control group). Then, the samples need to be chopped and grined, so the DNA and RNA can be extracted. After extraction, sequencing is done to DNA RNA and proteins, which leads to analysis of the data. By using instruments, the up regulation or down regulation of each patient’s DNA, RNA and protein can be clearly displayed, and this forms the data set. As described, each step has a high experimental error rate. Therefore, if this ready-made data is used, the accuracy of the experiment cannot be confirmed. Despite the of the limitation in data accuracy, using Multi Omics approach to analyse the data to detect the possible targets is still a very promising research direction while both results from our method included found targets of lung adenocarcinoma, which proved our method to be effective. This could offer an idea and enlightment for of analysis of future researches on the same topic. In the future, more and more people will get cancer in their early age, because this is already a global trend. Therefore, the demand of developing more targeted drugs to cope with this trend will increase as well. Moreover, targeted therapy can precisely attack on cancer cells, meanwhile having damage to normal civillians cells in patient’s body. Thus, this therapy for cancer will definitely be more of a treatment option compared to other conventional therapy (e.g chemotherapy), which proved the importance of the analysis of targets for lung adenocarcinoma using our method of multi-omics and bioinformatics. 5. Conclusion In a nutshell, we designed a possible approach to filter and locate targets for lung adenocarcinoma treatments and validated it’s functionality by proving that it is capable of finding possible genes interacting with cancer cells. We integrated the RNA expression with the protein expression level, and calculated z-score averages from data provided from a third party site after modelled into a normal distribution. Then we ranked RNA and gene expression in individuals and all luad patients as a whole to uncover possible target genes responsible or related to lung adenocarcinoma. The fact that we have discovered identified genes from our method proved that the method is completely practical, feasible and is able to provide useful information and shed light on future cancer research using bioinformatics. In addition, the coding in this method is not exclusive, which could be altered flexibly according to the demand of the researchers, as long as the central thesis is clear. 44 Proceedings of the 2nd International Conference on Modern Medicine and Global Health DOI: 10.54254/2753-8818/33/20240767 References [1] [2] [3] [4] [5] [6] [7] [8] Global Cancer Statistics 2020: GLOBOCAN Estimates of Incidence and Mortality Worldwide for 36 Cancers in 185 Countries Sung, H., Ferlay, J., Siegel, R. L., Laversanne, M., Soerjomataram, I., Jemal, A., & Bray, F. (2021). Global Cancer Statistics 2020: GLOBOCAN Estimates of Incidence and Mortality Worldwide for 36 Cancers in 185 Countries. CA Cancer J Clin, 71(3), 209-249. https://doi.org/10.3322/caac.21660 Liu Z, Zhao Y, Kong P, Liu Y, Huang J, Xu E, Wei W, Li G, Cheng X, Xue L, Li Y, Chen H, Wei S, Sun R, Cui H, Meng Y, Liu M, Li Y, Feng R, Yu X, Zhu R, Wu Y, Li L, Yang B, Ma Y, Wang J, Zhu W, Deng D, Xi Y, Wang F, Li H, Guo S, Zhuang X, Wang X, Jiao Y, Cui Y, Zhan. Integrated multi-omics profiling yields a clinically relevant molecular classification for esophageal squamous cell carcinoma. Cancer Cell. 2023 Jan 9;41(1):181-195.e9. doi: 10.1016/j.ccel2022.12.004. Epub 2022 Dec 29. PMID: 36584672 Schabath MB, Cote ML. Cancer Progress and Priorities: Lung Cancer. Cancer Epidemiol Biomarkers Prev. 2019 Oct;28(10):1563-1579. doi: 10.1158/1055-9965.EPI-19-0221. PMID: 31575553; PMCID: PMC6777859. Asada, K., Kobayashi, K., Joutard, S., Tubaki, M., Takahashi, S., Takasawa, K., Komatsu, M., Kaneko, S., Sese, J., & Hamamoto, R. (2020). Uncovering Prognosis-Related Genes and Pathways by Multi-Omics Analysis in Lung Cancer. Biomolecules, 10(4). https://doi.org/10.3390/biom1004052 Luan, M., Song, F., Qu, S., Meng, X., Ji, J., Duan, Y., Sun, C., Si, H., & Zhai, H. (2020). Multi omics integrative analysis and survival risk model construction of non-small cell lung cancer based on The Cancer Genome Atlas datasets. Oncol Lett, 20(4), 58. https://doi.org/10.3892/ol.2020.11919 Chen, M., Li, S., Liang, Y., Zhang, Y., Luo, D., & Wang, W. (2021). Integrative Multi-Omics Analysis of Identified NUF2 as a Candidate OncogenCorrelates With Poor Prognosis and Immune Infiltration in Non-Small Cell https://doi.org/10.3389/fonc.2021.656509 Lung Cancer. Front Oncol, 11, 656509. Dong, X., Leng, Y., Tian, T., Hu, Q., Chen, S., Liu, Y., & Shen, L. (2023). GALNT2, an O glycosylating enzyme, is a critical regulator of radioresistance of non-small cell lung cancer: evidence from an integrated multi-omics https://doi.org/10.1007/s10565-023-09825-6 [9] analysis. Cell Biol Toxicol. Zhang L, Parvin R, Chen M, Hu D, Fan Q, Ye F. High-throughput microfluidic droplets in biomolecular analytical system: A review. Biosens Bioelectron. 2023 May 15;228:115213. doi: 10.1016/j.bios.2023.115213. Epub 2023 Mar 8. PMID: 36906989. the [10] Daftuar, L., Zhu, Y., Jacq, X., & Prives, C. (2013). Ribosomal proteins RPL37, RPS15 and RPS20 regulate Mdm2-p53-MdmX network. PLoS One, 8(7), e68667. https://doi.org/10.1371/journal.pone.0068667
Football team
Club and game photos
KingLead_Sum
Lecture
LitClass
iGEM